Data Science and Global Health: Harnessing the Power of Secondary Data
- info058715
- Apr 4
- 5 min read
The integration of data science into healthcare has ushered in a new era of improving global health outcomes, especially in how we collect, analyze, and apply health data. While health data has traditionally been used to improve individual clinical care, there is a growing focus on how we can use both primary and secondary data sources to transform healthcare delivery on a broader scale. In this article, we explore the role of secondary data use in global health, its implications for high-resource and low- and middle-income countries (LMICs), and the challenges and opportunities it presents.
The Promise of Health Data in Improving Care Delivery
At the core of health data use is the improvement of clinical care. In high-resource settings, such as the United States, electronic medical records (EMRs) have become a central tool for enhancing healthcare delivery. By collating patient records, generating personalized alerts, and automating processes, EMRs help to streamline patient care and reduce errors. The federal government’s 2009 initiative in the U.S., which allocated $27 billion to encourage the adoption of EMRs in hospitals and clinics, exemplifies how health data systems can be scaled to improve healthcare services.
Despite the success of EMRs in individual clinical care, they offer a limited view of the broader factors that influence health at a population level. The real promise of health data lies in its ability to integrate diverse data sources, providing insights that can drive public health interventions and disease management strategies.
Secondary Data Use: A New Frontier for Health Improvement
Secondary data use refers to the analysis of health data beyond its original purpose, which, in the case of EMRs, is individual care delivery. Secondary data can be divided into two categories: direct and indirect use. Direct secondary data involves analyzing information directly sourced from health records, such as clinical audits or population health projects. This type of data helps inform future healthcare practices at both individual and population levels.
On the other hand, indirect secondary data involves using data not originally intended for health purposes, such as death certificates, billing data, criminal records, or even vehicle registration and commercial databases. When these disparate data sources are combined and analyzed, they can provide valuable insights into the health determinants of populations and inform targeted public health interventions.
Secondary data use makes it possible to address the broader factors influencing health that may not be directly related to clinical care, such as socioeconomic status, environmental factors, and access to healthcare services. This capability provides an essential mechanism for improving population health strategies, especially in resource-limited settings.
Data Science and Global Health in Low- and Middle-Income Countries
One of the most transformative impacts of data science is seen in low- and middle-income countries (LMICs), where technological advancements are often leapfrogging traditional infrastructure. The rapid proliferation of mobile phones in LMICs over the last two decades, without the need for fixed-line telephone networks, is a prime example of how technology can bypass older systems to drive innovation.
In global health, mobile phones have proven to be a valuable tool for indirect secondary data use. For example, researchers have tracked mobile phone use to better target malaria eradication efforts. A study by Buckee and colleagues showed that analyzing regional travel patterns of 15 million mobile phone users in Kenya revealed important information about malaria transmission routes. These insights allowed health organizations to focus mosquito control efforts in regions with the highest likelihood of transmission.
In LMICs, mobile health (mHealth) and electronic health (eHealth) projects often operate with different incentives than those in high-resource settings. Many such projects are government-run or operated by not-for-profit organizations with a focus on improving population health outcomes, in addition to individual care. However, these systems often face challenges such as lack of interoperability between platforms, which can hinder data analysis and linkage.
Challenges in Secondary Data Use for Health
The use of secondary data in healthcare, particularly in LMICs, is not without its challenges. One significant barrier is the difficulty in linking disparate data sources. Data integration is essential to unlock the full potential of secondary data, but it requires sophisticated technical expertise, specialized equipment, and regulatory frameworks to ensure data privacy and security.
For instance, the potential for secondary data to transform global health is hindered by a lack of standardized data collection and analysis systems. While mobile phones and other technologies offer low-cost avenues for data collection, the real value of secondary data comes from the ability to centralize and link multiple datasets. Achieving this requires interoperability between different systems, consistent data collection practices, and regulatory oversight, which are often lacking in LMICs.
Another challenge is the gap in analytical capacity in many LMICs. While mHealth solutions are widely adopted due to their ease of use and direct impact on individuals, the integration of health data at a population level is more complex. Overcoming this gap requires not only technical capacity but also investment in education and infrastructure to support data science and health analytics.
Opportunities in Global Health: A Case Study of India’s Aadhaar Program
Despite these challenges, there are promising examples of how secondary data can be used to improve global health outcomes. One such example is India's Aadhaar program, which began issuing biometric identification cards to its 1.2 billion citizens in 2010. Aadhaar uses biometric data like fingerprints and iris scans to uniquely identify individuals, offering a potential tool for monitoring health and social data.
By linking Aadhaar numbers to health records, India can track vaccination coverage, identify areas with low immunization rates, and target interventions more effectively. Secondary data analysis of de-identified health records could enable the identification of gaps in healthcare access, improving health outcomes across the country.
The Need for Robust Data Governance
As the use of secondary data in global health expands, the need for strong governance structures becomes even more critical. Data governance in the global health space has lagged behind the rapid growth of digital data and analytics. The United Nations' Global Pulse initiative, launched in 2009, aimed to foster the development of new analytical technologies to improve decision-making. However, data protection standards have not kept pace with advances in technology, and the current framework is based on outdated guidelines from 1990.
To maximize the benefits of big data in global health, more robust and contemporary data protection and governance mechanisms are required. These should include enforceable interoperability standards, clear guidelines for data sharing, and privacy safeguards. Establishing such frameworks will be essential to ensure that data is used ethically and effectively, particularly in LMICs where data protection and governance structures are often weak.
Conclusion: Building the Future of Global Health through Data Science
The potential for secondary data to revolutionize global health is immense, but it is not without its challenges. By linking diverse data sources and analyzing them on a population level, we can uncover insights that drive better health outcomes, particularly in resource-limited settings. However, to unlock this potential, significant investment in data infrastructure, technical expertise, and governance is required.
LMICs, with their rapidly evolving digital infrastructures, are uniquely positioned to leapfrog traditional systems and embed data science into their healthcare frameworks from the outset. By addressing the challenges of data integration, improving analytical capacity, and establishing strong data governance, we can harness the power of data to improve global health outcomes for generations to come.
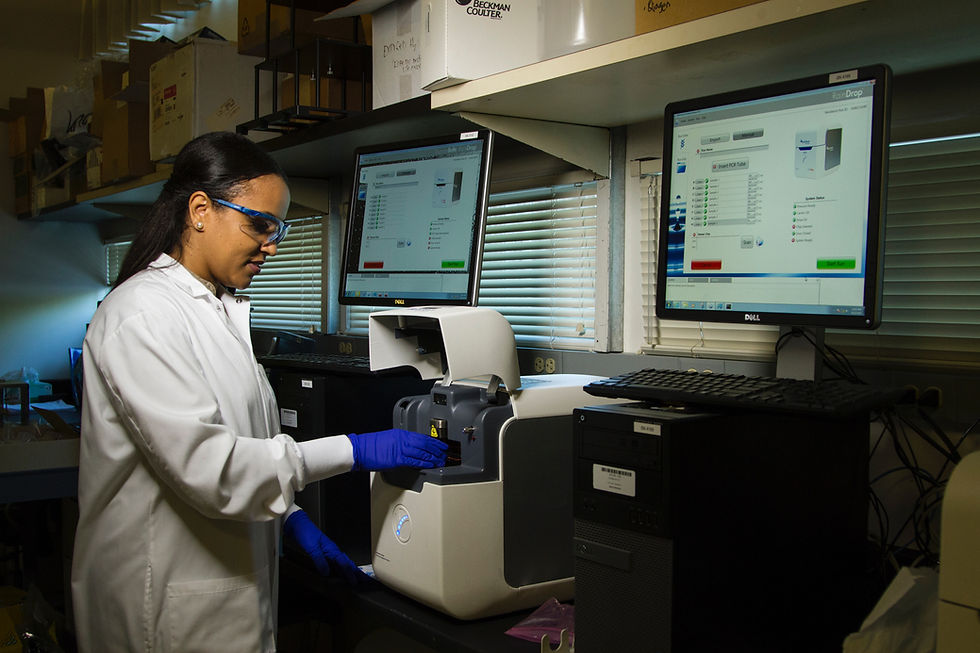
Comments